10 Benefits of AI in Data Science & How It’s Changing Industries
- SoftudeFebruary 11, 2025
- Last Modified onFebruary 11, 2025
Imagine a world where data analysis isn't a painstaking, manual process, but a dynamic, automated exploration fueled by intelligent algorithms. That world isn't a distant dream – it's the reality being shaped right now by the convergence of Artificial Intelligence (AI) and Data Science.

For years, data scientists have toiled, wrangling massive datasets, manually identifying patterns, and building predictive models. It’s a bit like searching for a single grain of sand on a beach; tedious and often overwhelming. Now, AI is stepping in as a powerful ally, automating repetitive tasks, uncovering hidden insights, and accelerating the entire data science lifecycle. Think of it as giving data scientists a super-powered magnifying glass, a high-speed data-crunching engine, and a crystal ball, all rolled into one.
In this blog we will dive deep into the top 10 benefits of integrating AI into data science, showcasing how this powerful combination is not just improving existing processes, but fundamentally reshaping industries from healthcare to finance and beyond. Get ready to explore how AI is transforming data from a static record of the past into a dynamic tool for predicting the future, making smarter decisions, and driving unprecedented innovation. Let's unlock the potential!
What is AI in Data Science?
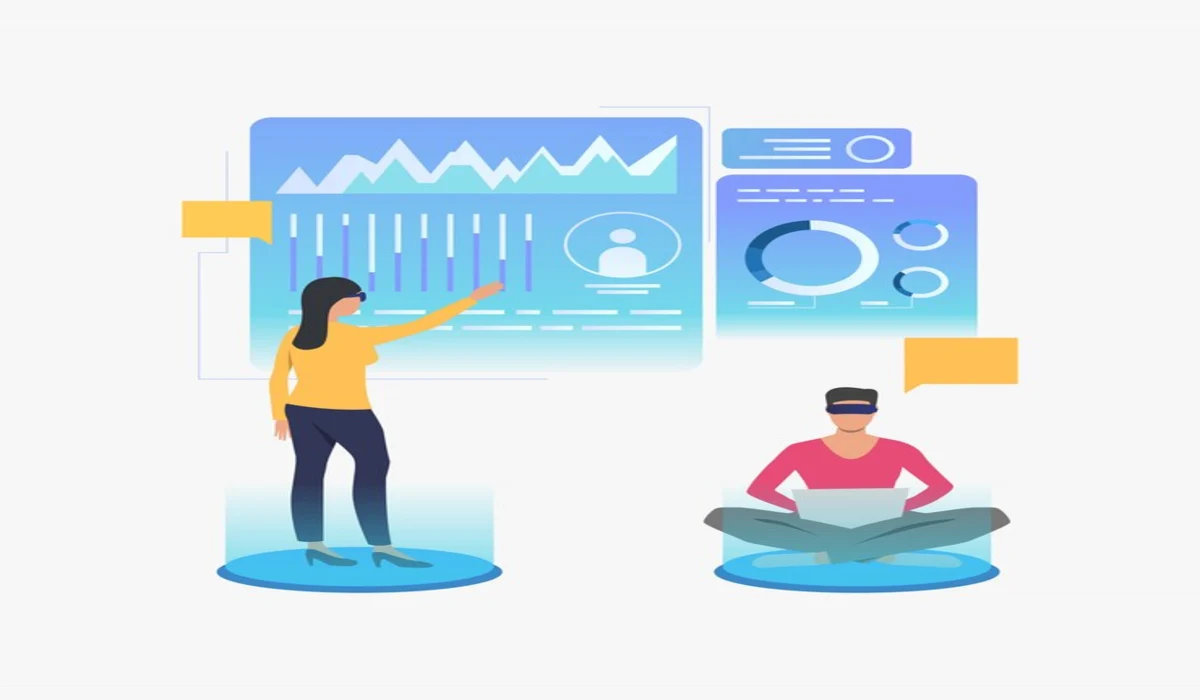
At its core, Artificial Intelligence refers to the development of systems that can mimic human cognitive abilities to perform tasks such as learning, problem-solving, and decision-making. This encompasses a wide range of techniques, but within data science, the most relevant are:
- Machine Learning (ML): Algorithms that learn from data without explicit programming. They identify patterns, make predictions, and improve their accuracy over time.
- Deep Learning (DL): A subset of machine learning that uses artificial neural networks with multiple layers to analyze data with greater complexity. Think of it as ML on steroids, capable of tackling more intricate problems.
- Natural Language Processing (NLP): Allows computers to understand, interpret, and generate human language. This is crucial for analyzing text data, understanding sentiment, and building conversational AI.
AI enhances data science by automating many steps in the data analysis pipeline. Traditional data science often involves manual data cleaning, feature engineering, and model selection – all time-consuming processes. AI algorithms can automate these tasks, allowing data scientists to focus on higher-level strategic thinking and problem-solving.
Top 10 Benefits of AI in Data Science
1. Automation of Data Collection and Preprocessing
Gathering and preparing data can be a major bottleneck in any data science project. AI-powered tools can automate data extraction from various sources, including websites, databases, and APIs. Furthermore, AI algorithms can automatically clean and transform data, handling missing values, outliers, and inconsistencies with minimal human intervention. Imagine automatically scraping product reviews from e-commerce sites, cleaning the text, and structuring it for sentiment analysis – all without writing a single line of manual code.
Example:
A retail company collects customer feedback from social media, emails, and product reviews. AI-powered tools like web scrapers and sentiment analysis engines automatically extract, clean, and categorize this data to identify customer preferences without manual intervention.
2. Enhanced Data Analysis and Visualization

AI algorithms excel at identifying complex patterns and anomalies in large datasets that might be invisible to the human eye. They can sift through massive amounts of information, uncover hidden correlations, and generate insightful visualizations that tell a compelling story. For example, AI can analyze customer purchase history to identify distinct customer segments, visualize their buying behavior, and tailor marketing campaigns accordingly. Think of it as having an AI-powered detective that uncovers hidden clues within your data.
Example:
A marketing team uses AI-driven analytics tools like Tableau or Power BI to analyze customer demographics and purchase patterns. The AI generates interactive heatmaps and graphs, helping businesses tailor their ad campaigns effectively.
3. Predictive Modeling and Forecasting
AI algorithms are the workhorses behind accurate predictive models. They can learn from historical data to forecast future trends, predict customer behavior, and optimize business decisions. Whether it's predicting sales, forecasting demand, or assessing risk, AI-powered predictive models can provide valuable insights that drive better outcomes. For instance, retailers use AI to predict which products will be in high demand during the holiday season, allowing them to optimize inventory and avoid stockouts.
Example:
E-commerce platforms like Amazon use AI-powered predictive models to analyze previous purchase history and suggest products that customers are likely to buy next, increasing sales and customer retention.
4. Improved Feature Engineering
Feature engineering – the process of selecting and transforming relevant variables – is a critical step in building effective machine learning models. AI can automate this process by automatically identifying and selecting the most important features from a dataset. It can also create new features by combining existing variables, leading to improved model performance. Imagine an AI algorithm automatically identifying the key factors that influence customer churn, allowing you to focus your retention efforts on the most at-risk customers.
Example:
A fintech company uses AI to automatically identify the most important variables affecting loan approvals, such as income stability, spending habits, and credit history, optimizing their machine-learning model for risk assessment.
5. Real-time Data Processing
In today's fast-paced world, the ability to process data in real-time is becoming increasingly important. AI enables real-time analysis and processing of streaming data, allowing organizations to respond quickly to changing conditions. For example, financial institutions use AI to monitor transactions in real-time, detect fraudulent activities, and prevent financial losses. Think of it as having an AI-powered security guard that constantly monitors your data and flags any suspicious activity.
Example:
Stock trading platforms use AI to analyze market trends in real-time, enabling traders to execute profitable trades based on instant insights rather than delayed manual analysis.
6. Natural Language Processing (NLP) for Data Insights
.webp)
A huge amount of valuable data is locked away in text format – customer reviews, social media posts, emails, and more. NLP techniques unlock this data by enabling computers to understand, interpret, and extract insights from human language. Sentiment analysis, topic modeling, and text summarization are just a few of the NLP applications that can provide valuable insights into customer opinions, market trends, and competitive intelligence. For instance, a company can use NLP to analyze customer reviews and identify areas where its products or services need improvement.
Example:
A customer service chatbot analyzes thousands of support tickets using NLP to detect common issues, helping businesses proactively address customer concerns by improving FAQs and product documentation.
7. Anomaly Detection and Fraud Prevention
AI algorithms are adept at identifying unusual patterns and anomalies in data, making them ideal for detecting fraud and preventing other types of malicious activity. They can learn the normal behavior of a system or process and flag any deviations from that norm. This is particularly useful in industries like finance, healthcare, and insurance, where fraud is a significant concern. For example, AI can analyze credit card transactions to identify suspicious patterns and prevent fraudulent purchases.
Example:
Banks use AI-powered fraud detection systems that flag unusual transaction patterns, such as a sudden high-value transfer from an unfamiliar location, preventing potential fraud in real-time.
8. Hyper-personalization
Customers today expect personalized experiences. AI enables businesses to deliver hyper-personalized experiences by analyzing individual customer data and tailoring products, services, and marketing messages to their specific needs and preferences. Recommendation systems, targeted advertising, and personalized content are all powered by AI. Think of Netflix suggesting movies you might like based on your viewing history, or Amazon recommending products based on your past purchases.
Example:
Netflix recommends TV shows and movies based on a user’s watch history and preferences, ensuring a customized viewing experience that keeps users engaged.
9. Automated Decision-Making
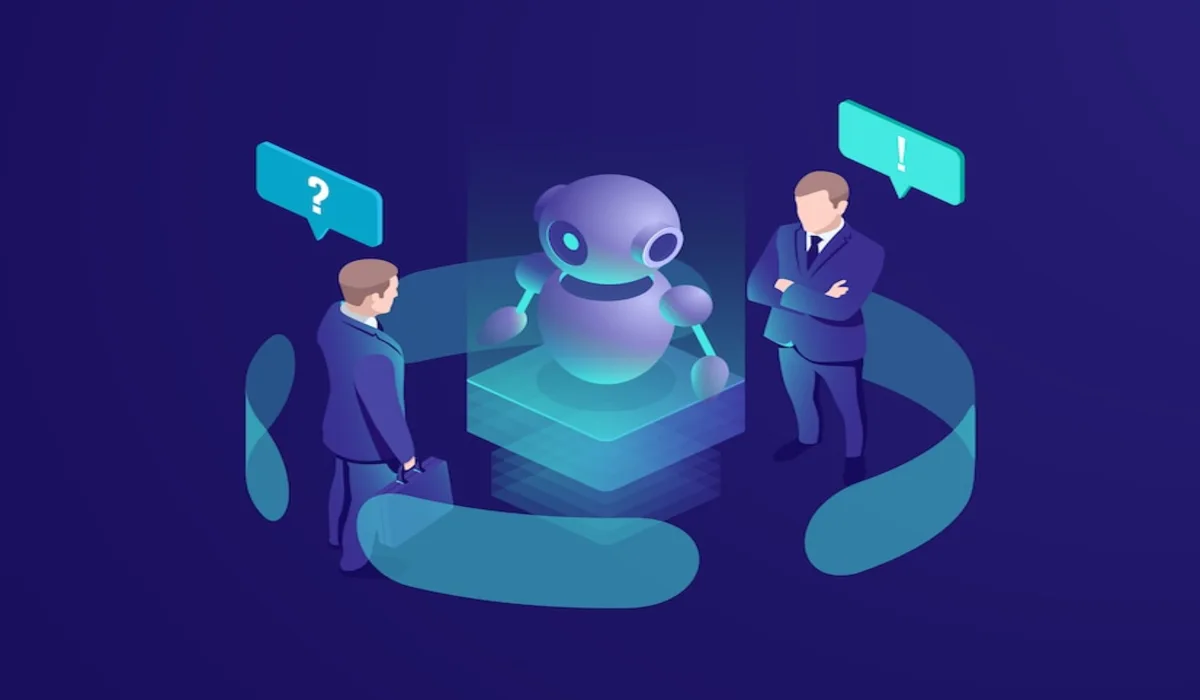
AI systems can make autonomous decisions based on data analysis, freeing up human employees to focus on more strategic tasks. Algorithmic trading, autonomous vehicles, and automated customer service are just a few examples of how AI is enabling automated decision-making. For instance, in financial markets, AI-powered trading algorithms can execute trades automatically based on predefined rules and market conditions.
Example:
Self-driving cars use AI to analyze traffic conditions, pedestrian movements, and road signs, making split-second decisions to navigate safely without human intervention.
10. Scalability and Efficiency
AI can handle large volumes of data and automate complex tasks, making data science workflows more scalable and efficient. Cloud-based AI platforms provide access to vast computing resources and pre-built AI models, allowing organizations to scale their data science capabilities without significant investments in infrastructure. Think of it as having access to a virtually unlimited pool of computing power that can be scaled up or down as needed.
Example:
Cloud-based AI platforms like Google Cloud AI and AWS allow businesses to process massive datasets without investing in expensive on-premise infrastructure, scaling up operations effortlessly.
Conclusion
AI is transforming data science by automating processes, enhancing analytics, and driving smarter decisions. Businesses leveraging AI gain a competitive edge through predictive modeling, anomaly detection, and intelligent automation.
At Softude, we help organizations unlock AI’s full potential with data science and machine learning consulting. Whether you need automated workflows, advanced analytics, or AI-driven insights, we provide tailored solutions to maximize your data’s value.
Ready to integrate AI into your data strategy? Explore our AI & data science consulting services.
Liked what you read?
Subscribe to our newsletter